여론조사 함정, 과대표집, 과소표집, 오버샘플링, 언더샘플링, Over-Sampling, Under-Sampling
과대표집, 과소표집
오버샘플링, 언더샘플링, Over-Sampling, Under-Sampling
자료 집합의 비율을 조정하는 것이다. 특정 클래스가 실제 비율보다 과도하게 많이 표집되었다면 오버샘플링(과대표집, 誇大標集, 과다표집, overampling), 과도하게 적게 표집되었다면 언더샘플링(과소표집, 誇少標集, undersampling)이라고 한다. 불균형한 데이터의 경우, 실제 성능을 높이기 위해 비율을 조정해서 일부러 많이 들어있는 종류는 오버샘플링하고, 적게 들어있는 종류는 언더샘플링을 해야 하는 경우도 있다.
1. 불균형 자료
Sampling에 대해 알기 위해선 먼저 불균형 자료에 대해 알아야한다.
불균형 자료는 목표변수의 빈도(분포)가 한쪽으로 치우친 자료를 의미한다.
쉬운 이해를 위해 예를 들어보면 제조 공정의 양품/불량 에서의 불량 데이터, 암 판정의 음성/양성 에서의 양성 데이터 등을 생각해볼 수 있다.
이런 불균형 데이터를 활용한 모델은 정확도는 높을 수 있지만 재현율이 낮아 모델의 성능이 저하될 수 있다.
2. 불균형 자료 처리
불균형 데이터 처리를 위해서는 Over-Sampling과 Under-Sampling이 있다.
1). Over-Sampling
다수의 자료는 모두 선택하고, 무작위 추출로 소수의 자료를 복제한다.
정보 손실은 방지할 수 있지만 복제된 특정 자료를 원래 데이터에 추가하면서 Over Fitting이 될 가능성이 있다.
따라서 원본 데이터의 값을 아주 조금만 변경하여 복제하는 것이 필요하다.
대표적인 방법으로 SMOTE(Synthetic Minority Over-sampling Technique)가 있다.
동일한 데이터를 단순히 증식시키지 않기 위해 개별 데이터들의 KNN을 활용하여 K개 이웃들의 차이를 일정 값으로 만들어 기존 데이터와 약간의 차이를 두는 방식이다.
2). Under-Sampling
무작위로 다수의 자료 중 일부를 선택하고, 소수의 자료 전체를 선택한다.
즉, 유의한 데이터만 남기고 나머지는 활용하지 않는 것을 의미한다.
데이터의 소실이 매우 커서 다수 자료의 중요한 정보가 손실될 우려가 있어 실제 분석에서 Over-Sampling을 더 많이 활용하고 있다.
3. 분석 시 활용방안
모델링을 진행하기 전 바로 실시하기 보다 기본적인 모델로 진행한 후 Recall(재현율) 값을 확인해보고 너무 낮을 때 적용하는 것이 좋을 것 같다.
여론조사의 함정... 과다표집이 만든 민심 착각과 국민의힘의 딜레마
ARS 조사와 정치적 관심도, 왜곡된 여론의 원인
보수층 결집이 민심의 전부인가? 중도층의 역할을 간과한 위험
과거 탄핵 정국의 실수 재현되나, 국민의힘의 대선 전략 논란
최근 여론조사 결과에서 특정 정치 성향의 지지율이 과도하게 높게 나타나는 '과다표집' 현상이 논란의 중심에 섰다. 윤석열 대통령 체포 이후 보수층 지지자들이 여론조사에 적극 응답하면서 국민의힘의 지지율 상승세가 두드러졌으나, 이를 맹신하는 지도부의 태도가 또 다른 전략적 실수를 초래할 가능성이 제기되고 있다.
과다표집 현상은 조사 방법, 응답률 저하, 정치적 관심도의 차이 등 복합적인 요인에 의해 발생하며, 이번 여론조사에서도 이러한 문제가 고스란히 드러났다.
여론조사 방법론을 보면 자동응답시스템(ARS)을 활용한 조사의 경우, 정치적 관심이 높은 응답자들이 적극적으로 참여하는 경향이 뚜렷하다. 박종희 서울대 정치외교학부 교수는 "ARS 조사의 특성상 정치에 관심이 많은 집단의 의견이 과대 대표될 가능성이 높다"고 분석하며, 이는 부동층의 목소리가 상대적으로 배제되는 결과를 초래한다고 지적했다.
실제로 최근 전국지표조사(NBS)에서도 보수층 결집이 여론조사 응답률에 영향을 미쳐 국민의힘이 더불어민주당을 오차범위 내에서 앞섰다. 국민의힘은 35%, 더불어민주당은 33%를 기록했으나, 전문가들은 이러한 결과가 민심 전체를 대변하지 못할 수 있다고 경고한다.
엄경영 시대정신연구소장은 "윤 대통령 체포로 인해 보수층이 결집하면서 여론조사 응답률이 높아졌고, 이는 실제 민심보다 보수층의 지지율이 과대 대표된 결과로 이어졌을 가능성이 크다"고 분석했다. 특히, NBS 조사에서 보수 성향 응답자가 진보 성향 응답자보다 많은 비율을 차지하며 이러한 경향을 강화한 것으로 나타났다. 조사에서 '진보'는 257명, '보수'는 344명으로 집계되어 체감 민심과 비교했을 때 왜곡의 여지가 있다는 비판이 제기된다.
이와 함께 정치적 관심도와 조사 시점의 영향도 과다표집 논란의 주요 요인으로 꼽힌다. 정치적 이슈나 상황에 따라 특정 성향의 지지층이 여론조사에 적극적으로 응답하게 되면 결과적으로 편향된 여론이 나타날 가능성이 높다.
이는 과거 박근혜 전 대통령 탄핵 정국에서도 유사한 형태로 나타났던 현상으로, 당시 보수층의 결집이 여론조사 지지율을 끌어올리는 효과를 보였으나 실제 선거에선 패배로 이어졌다.
국민의힘 지도부는 최근 여론조사 결과를 근거로 대선 전략을 조정하고 있으나, 당 내부에서도 이에 대한 우려가 커지고 있다. 수도권 출신 일부 의원들은 "여론조사 결과를 맹신해 강성 지지층에 의존하는 전략은 대선 본선에서 큰 실패를 초래할 수 있다"고 경고했다.
이들은 탄핵 이후 변동하는 민심에 효과적으로 대응하기 위해 중도층과 청년층의 표심을 잡는 현실적이고 유연한 전략이 필요하다고 주장했다. 반면, 당내 강경파는 여론조사 결과를 지지층의 결집 신호로 해석하며, 강경 노선을 이어가야 한다고 주장하고 있다.
또한, 탄핵 정국에서 국민의힘이 보수층의 지지율 상승에만 의존할 경우, 과거와 같은 실수를 반복할 수 있다는 지적이 나온다. 전문가들은 여론조사가 순간적인 흐름일 뿐이며, 실제 선거 결과와는 다를 수 있다는 점을 강조하며, 장기적 관점에서 다양한 민심을 반영한 전략이 필요하다고 조언하고 있다.
특히, 국민의힘이 탄핵 정국 이후 윤 대통령과의 거리두기 전략을 포함해 보다 포괄적인 접근을 통해 중도층을 포섭하지 못한다면, 대선에서 불리한 상황을 맞을 가능성이 높다는 전망도 나오고 있다.
한편, 여론조사의 신뢰성을 높이기 위해 조사 방법의 다양화와 표본의 대표성 확보가 중요하다는 목소리가 높다. 응답률을 높이기 위한 제도적 보완과 함께 조사 결과 해석 시 과다표집 문제를 인지하고 신중히 접근해야 한다는 지적이다. 이번 사태를 계기로 국민의힘이 여론조사에만 의존한 단기적 전략에서 벗어나, 탄핵 이후 상황을 대비한 유연한 전략을 통해 민심의 변화를 읽어내는 능력을 기르는 것이 절실히 요구된다.
결국, 윤 대통령의 체포로 인한 보수층 결집과 여론조사 과다표집의 현상이 국민의힘 내부 전략과 대선 판도에 미칠 영향을 신중히 분석해야 할 시점이다.
민심의 변동성과 복합적인 사회적 맥락을 고려하지 않은 전략은 대선이라는 중요한 승부에서 또 한 번의 패배로 이어질 수 있다. 탄핵 정국 이후 예상되는 변수들에 대비한 국민의힘의 대응이 향후 정치 지형을 결정짓는 중요한 분기점이 될 것이다.
더불어민주당은 4·10 총선 사전투표 개시를 하루 앞둔 4일 각종 여론조사에서 도드라진 정권심판 우세 경향에도 안심하지 못하고 있다. 일부 여론조사 전문가들은 진보 성향 응답자가 과대표집됐을 가능성을 제기했다. 20·30세대, 무당층의 표심도 오리무중이다. 이재명 민주당 대표는 여론조사가 조작됐을 가능성까지 제기하고 나섰다.
이 대표는 이날 부산 영도구 유세에서 “앞으로 온갖 해괴한 여론조사가 나올 것이고 (국민의힘에서) ‘박빙 지역에서 지면 100석이 무너질지 모른다’는 협박 아닌 협박, 공갈 아닌 공갈이 많이 나올 것”이라며 “속아서는 안 된다. 여론조사는 앞으로 완전히 외면하라”라고 지지자들에게 당부했다. 이 대표는 전날 즉석 유튜브 방송에서도 “터무니없는 조작에 가까운 여론조사들이 이제 막 나올 것”이라며 “하루아침에 지지율이 20%포인트씩 바뀌는 황당무계한 여론조사도 나왔다. 여론조사는 정상적으로 하면 500명 샘플을 해도 오차가 8.8%포인트를 넘을 수 없는데 15%포인트씩 변동한다. 그럼 둘 중 하나는 가짜인 것”이라고 설명했다.
일각에서는 최근 일부 여론조사에서 진보 유권자가 과표집됐을 가능성이 있다고 본다. 최병천 신경제연구소장은 통화에서 “한국 유권자 지형상 보수가 진보보다 일반적으로 많은데, 일부 지역 여론조사에서 보수 응답자가 진보보다 적게 나오고 있다”고 설명했다.
한국갤럽이 지난 3월 한 달간 전국 유권자 4004명을 대상으로 한 전화조사원 인터뷰 조사 (95% 신뢰수준, 표본오차 ±3.1%포인트)에서 스스로 보수라고 밝힌 응답자는 32%, 중도는 39%, 진보는 28%였다. 보수층이 진보층보다 4%포인트 많다. 부산·울산·경남 지역에서는 보수 38%, 중도 29%, 진보 24%로 보수층 비율이 진보층보다 14%포인트 더 많다. 2021년 8월 이후 보수가 진보보다 많은 상태가 이어지고 있다.
그런데 부산 등 일부 격전지 지역구 여론조사에서 진보층이 보수층과 엇비슷한 응답률을 보였다. 부산일보와 부산MBC가 한국사회여론연구소(KSOI) 의뢰해 지난 1~2일 부산 연제구 유권자 506명을 대상으로 진행한 자동응답(ARS) 조사(95% 신뢰수준, 표본오차 ±4.4%포인트)에서 스스로 보수라고 밝힌 응답자는 27.7%, 중도 35.0%, 진보 24.5%로 집계됐다. 같은 조사에서 노정현 진보당 후보는 56.7%를 받아 김희정 국민의힘 후보(37.5%)를 오차범위 밖인 19.2%포인트 앞섰다.
일부 전문가들은 여론조사 응답률 저하 경향을 진보 과표집 배경으로 분석하고 있다. 정한울 정치학 박사는 이날 사회관계망서비스(SNS)에 최근 2년 사이 선거여론조사 협조율은 21.9%에서 13.5%까지 떨어졌고, AAPOR(국제 기준 응답률=접촉률×응답률) 기준 응답률도 같은 기간 6.1%에서 4.5%로 낮아졌다고 밝혔다. 정 박사는 “문제는 응답률이 낮은 조사일수록 민주당 지지율이 과대대표된다는 점”이라며 “현재 민주당 지지율에 거품이 껴 있을 수 있음을 의미한다”고 짚었다.
20·30세대, 무당층 최종 투표율 변수도 남아 있다. 중앙선거관리위원회가 한국갤럽에 의뢰해 지난달 18~19일 전국 유권자 1500명을 대상으로 진행한 ‘22대 총선 관련 유권자 의식조사’(95% 신뢰수준에 표본오차 ±2.5%포인트)를 보면 18~29세의 총선 관심도는 56.8%로 21대 총선 때(64.3%)보다 7.5%포인트 떨어졌다.
한국갤럽이 지난달 26~28일 유권자 1001명을 대상으로 한 전화인터뷰 조사(95% 신뢰수준, 표본오차 ±3.1%포인트)에서 20대와 30대 중 무당층 비율은 각각 38%와 29%였다. 같은 기관의 4년 전 21대 총선 직전 조사(2020년 4월 7~8일) 때보다 20대와 30대 무당층 비율이 각각 6%포인트, 5%포인트 늘어났다. 최 소장은 “이번 총선 투표율이 4년 전 기록인 66.2%보다 떨어질 가능성도 있다”며 “투표율은 정치권에 대한 기대와 열망이 있어야 오르는데, 20·30세대 입장에서 보면 윤석열 정부가 실정했지만 4년 전 총선 때보다 정치권에 대한 기대가 적을 수도 있다”고 분석했다.
한병도 민주당 선대위 전략본부장은 이날 서울 여의도 당사에서 기자들과 만나 “여론조사에 고연령층이 많이 응답하기에 세대별 응답도 잘 봐야 한다”며 “여론조사 수치가 몇 % 나왔으니 이기리라 판단하는 오류를 범하기 굉장히 쉽다. (야권) 170~180석 너무 믿지 말라”고 말했다.
In an imbalanced dataset, the classes are not evenly distributed but rather highly skewed. The skewness is not particularly the issue here but since one of the classes (referring binary classification) is overly frequent, it will affect the learning mechanism of the Machine Learning algorithm while the business is more interested in the occurrence of the rare class.
I understand that my introductory lines should be explained with a little more depth. The risk in an imbalanced dataset is that, the Machine Learning algorithm may actually ignore the rare class cases and incorrectly classify as frequent class. Let me take an example (fig-1) which is a popular scenario i.e., identifying fraudulent transactions.
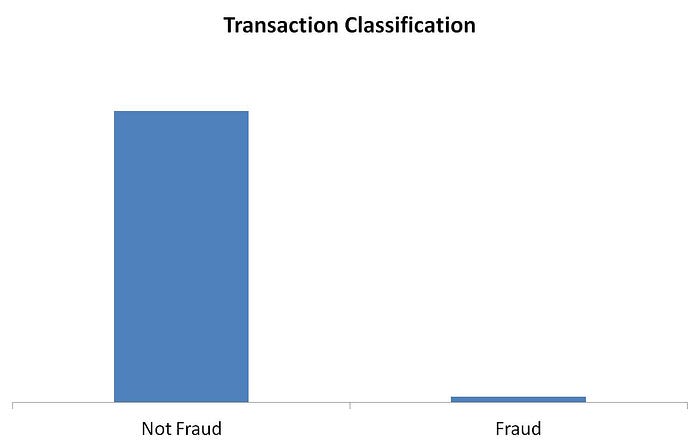
(fig-1) image source: self created
As you may understand, it is unlikely to have significant fraudulent transactions in any financial structure. However, it is of utmost interest for the business to effectively identify the fraudulent transaction whenever they occur. You really don’t want your Machine Learning algorithm to incorrectly detect any fraudulent transaction as a valid else it will be a catastrophe for the business. That’s where the challenge of an imbalanced dataset lies.
If I am to explain it more from a technical standpoint, the decision function of a Machine Learning algorithm is hugely impacted by the training samples. Greater the imbalance ratio, the decision function favors the class that is more abundant i.e., the majority class.
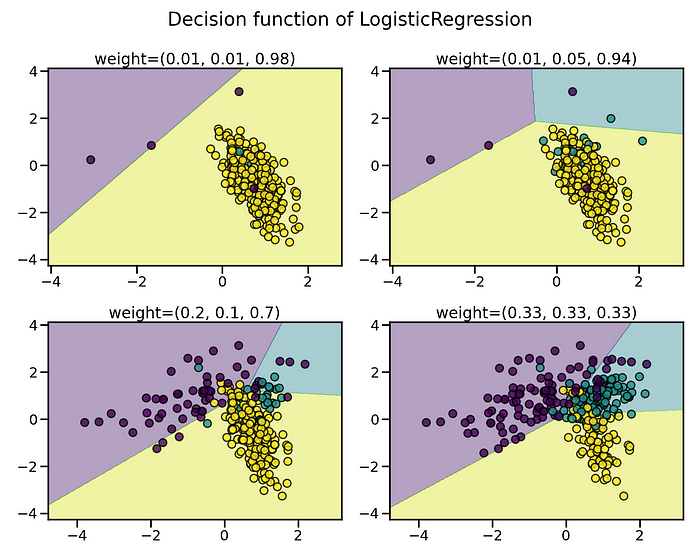
image source: https://imbalanced-learn.org/
Approach
The idea to combat the challenge of imbalanced data is random sampling. Now random resampling can be done in couple of ways (i) Oversampling and (ii) Undersampling
Oversampling — Generate new samples for the class which is under-represented.
Undersampling — Remove samples from the class which is over-represented.
Both oversampling & undersampling are ways to infuse bias where you take more samples from one class than the other to neutralize the effect of the imbalance that is either already present in the data or likely to be developed if the samples were taken purely at random.
Random sampling is a naive way to perform sampling as it completely ignores the inner artifacts of the data.
Setting the stage
We have already spoke a descent amount about the the imbalance data problem, now lets focus on the solution. Here in this discussion, we will use imbalanced-learn API which was started back in 2014, fully compatible with scikit-learn as well. Visit imbalanced-learn official website for the installation guidelines and entire API documentation.
#Imports
from collections import Counter
from sklearn.datasets import make_classification
from imblearn.under_sampling import RandomUnderSampler
from imblearn.over_sampling import RandomOverSampler
#Creating a dataset with 2 classes
X, y = make_classification(n_samples=15000, n_classes=2,
weights=[0.99, 0.01], flip_y = 0)
#class distribution
print(Counter(y))
#Output: Counter({0: 14850, 1: 150})
#class 0 has 14850 rows wheres class 1 has only 150 instances
I have created a binary classification dataset with a high imbalance ratio of 99% for majority class. Before we dig deep into various sampling techniques, lets first try to grab the idea of resampling.
#creating the instance for random over sampler
randomOverSampler = RandomOverSampler()
#performing resampling
X_over, y_over = randomOverSampler.fit_resample(X, y)
Counter(y_over)
#Output: Counter({0: 14850, 1: 14850})
The over sampler here created more samples from within the under represented class — fairly simple.
As the over sampler creates copies of the minority class, as a result over sampling technique in a way increases the probability for over-fitting.
The under sampler does just the opposite to the over sampler. It removes the instances from the majority class while resampling from the original dataset.
#creating the instance for random over sampler
randomUnderSampler = RandomUnderSampler()
#performing resampling
X_under, y_under = randomUnderSampler.fit_resample(X, y))
Counter(y_under)
#Output: Counter({0: 150, 1: 150})
The under sampler removes huge amount of rows from the majority class and hence it poses serious threat for under-fitting.
Different Sampling Techniques
For majority of the cases, oversampling is preferred more than undersampling. Removing data points is not ideal as it may carry significant piece of information. We will be focusing some of the popular sampling techniques pertaining to imbalanced data classification.
1. Synthetic Minority Oversampling Technique (SMOTE)
Instead of creating copies of existing instances of minority class like RandomOverSampler, SMOTE generates new illustrations through interpolation.
The way SMOTE creates synthetic data point is actually a sequence of few steps.
(i) Select a random instance within the minority class.
(ii) Identify k nearest neighbors (k=5 by default for KNN) for the randomly selected data point.
(iii) Select one of those neighbors for the synthetic data point to be created.
(iv) Calculate the distance vector between the data point and its neighbor.
(v) Multiply a random number between [0,1] to get the synthetic data point.
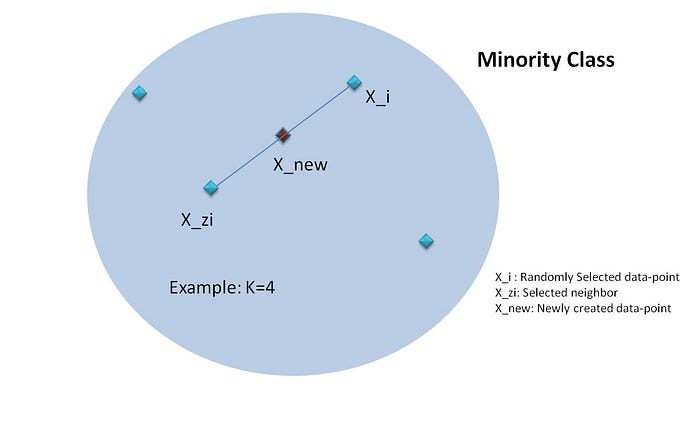
SMOTE Mechanism: self created
from imblearn.over_sampling import SMOTE
#creating the instance for SMOTE
smote = SMOTE()
#Resampling with SMOTE
X_smote, y_smote = smote.fit_resample(X, y)
Counter(y_smote)
#Output: Counter({0: 14850, 1: 14850})
2. Adaptive Synthetic (ADASYN)
ADASYN works similar to SMOTE i.e., this algorithm as well looks to create synthetic instances of the minority class. There is one difference though. The synthetic instances are generated for the samples which are not in homogeneous group or difficult to learn.
Here are the sequence of steps that ADASYN follows:
(i) Calculate the number of synthetic instances to generate (N_syn). Let N_maj & N_min denoting majority and minority classes respectively then
N_syn = (N_maj — N_min)*b where b is the class ratio. if b=1 then we will be seeing a perfectly balanced dataset.
(ii) Find the k nearest neighbors and calculate the d value where
d_i = (#majority/k). d_i value indicates the affluence of the majority class. Greater the value of d_i, more it will be difficult to learn.
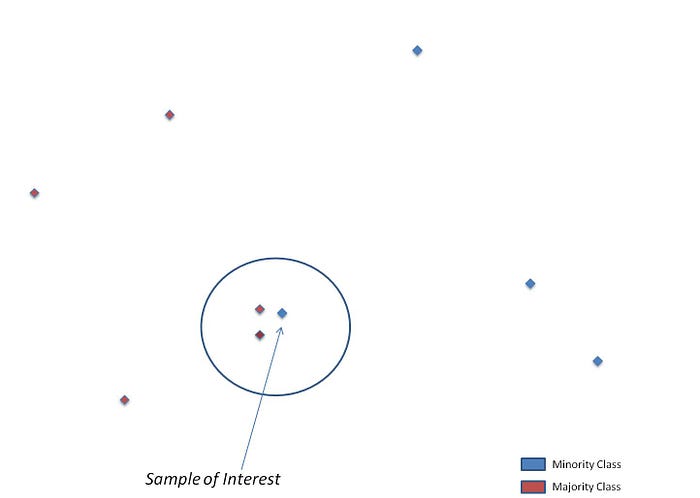
Choosing the minority class instance with most majority class neighbors
(iii) Now, follow the similar steps as SMOTE to calculate the distance vector, then take a random vector between [0,1] and accordingly create the synthetic instance.
from imblearn.over_sampling import ADASYN
#creating the instance for ADASYN
adasyn = ADASYN()
#Resampling with SMOTE
X_adasyn, y_adasyn = adasyn.fit_resample(X, y)
Counter(y_adasyn)
#Output: Counter({0: 14850, 1: 14829})
There are also many more sampling techniques where both oversampling and undersampling techniques are combined — :
i) SMOTE & Tomek Links (SMOTETomek)
ii) SMOTE & Edited Nearest Neighbors (SMOTEENN)
Let me show the python code snippet for both of these.
#SMOTE & Tomek Links
from imblearn.combine import SMOTETomek
from imblearn.under_sampling import TomekLinks
smote_tomek = SMOTETomek(tomek=TomekLinks(sampling_strategy='majority'))
X_smotomek, y_smotomek = smote_tomek.fit_resample(X, y)
#SMOTE & ENN
from imblearn.combine import SMOTEENN
smoteenn = SMOTEENN()
X_smotenn, y_smotenn = smoteenn.fit_resample(X, y)
Conclusion
There are innumerable number of scenarios where you will encounter imbalanced dataset problem. Some of the most common are — detect fraudulent transaction, churn prediction, anomaly detection etc. I have discussed the sampling techniques in this article that are helpful for better detection of minority class in a classification problem.
Accuracy is not a good measure any classification problem which is imbalanced. Hence, you must check recall, precision & f1 score as model metrics. However, it all depends on your business case whether to maximize recall or anything else.